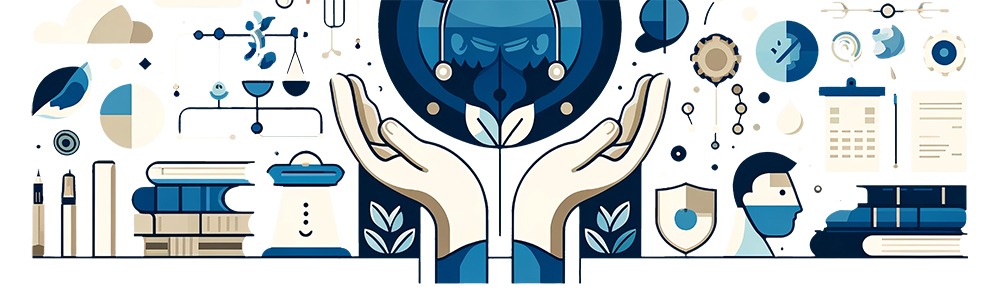
Artificial Intelligence News
with a Social Focus
Weekly Publication | CC BY-NC 4.0 | Dr. Diego Bonilla
Introduction
In an era where artificial intelligence is rapidly transforming education and social structures, the need for systematic analysis of these changes has never been more critical. AI News Social addresses this need through a methodologically robust approach that combines over 300 specialized search queries across ten distinct categories, ranging from AI literacy to social justice applications.
This weekly publication employs Paul and Elder's critical thinking framework to ensure thorough, balanced coverage of AI's impact on higher education and society. As an open educational resource designed specifically for higher education faculty, it serves as a crucial bridge between technological advancement and practical implementation in academic settings.
Through careful curation and synthesis of information from academic articles, university pages, news media, and online resources, the publication provides comprehensive insights into AI's potential, risks, and societal implications. The methodology detailed below reflects our commitment to delivering current, relevant, and valuable content that supports faculty in making informed decisions about AI integration while remaining mindful of ethical considerations and social justice implications. By combining advanced search techniques, expert analysis, and multi-language accessibility, this systematic approach ensures that educators have access to the tools and knowledge necessary to navigate the complex landscape of AI in higher education.
Methodology
Topics and Categorization
AI News Social uses multi-step approach to gathering information on artificial intelligence (AI) in higher education and social justice contexts. It is based on search queries that are structured to capture a wide range of AI applications, impacts, and considerations across several key categories:
AI Literacy (≈33 queries) This category focuses on understanding and implementing AI in educational settings. It covers topics such as: ChatGPT in academia, Ethical concerns and copyright issues, AI tools for instruction and assessment, and AI literacy for faculty development.
AI Tools (≈21 queries) These queries explore specific AI applications in education, including: Active learning and adaptive learning systems, Blended learning environments, Course management and instructional design, and Virtual reality and immersive learning technologies.
Education (≈60 queries) This broad category encompasses various aspects of AI in education: Curriculum design and syllabus generation, Student assessment and performance evaluation, Personalized learning and adaptive pedagogy, and Educational content creation and delivery.
Education EDU (≈60 queries) Similar to the Education category, but specifically targeting .edu domains, these queries focus on: AI applications in higher education institutions, Academic counseling and student support, Administrative automation in universities, and Research and educational technology advancements.
Education News (≈21 queries) This category aims to capture current developments and news articles related to: AI in higher education policies, Innovations in educational AI, Impact of AI on teaching and learning practices, and Ethical considerations and challenges in educational AI.
Education Scholar (≈60 queries) These queries target scholarly research on AI in education, covering: AI-assisted assignment creation and assessment, Intelligent tutoring systems, Learning analytics and predictive models, and AI ethics in educational research.
Social Justice (≈20 queries) This category explores the intersection of AI and social justice issues: Accessibility enhancement and inclusive design, Bias detection and mitigation, AI for climate justice and sustainable solutions, and AI in human rights monitoring and advocacy.
Social Justice EDU (≈20 queries) Similar to the Social Justice category, but focused on .edu domains, these queries investigate: AI applications for equity in educational settings, Addressing racial and gender biases in education through AI, and AI-driven solutions for poverty reduction and healthcare equity in academic contexts.
Social Justice News (≈21 queries) This category aims to capture current news and developments in AI and social justice: AI applications in civic engagement and public safety, Ethical governance and fair employment practices, and AI-driven solutions for climate justice and sustainable development.
Social Justice Scholar (≈20 queries) These queries target scholarly research on AI and social justice: AI in community-driven development, Fair resource distribution and poverty reduction strategies, and AI applications in legal justice advancement and human rights monitoring.
The main objectives of the Topics and Categorization are:
Comprehensive Coverage: The queries demonstrate a thorough approach to exploring AI in education and social justice, covering technological, pedagogical, ethical, and societal aspects.
Multi-faceted Approach: By categorizing queries into general, EDU-specific, news, and scholarly research, the method ensures a well-rounded perspective on each topic.
Interdisciplinary Focus: The inclusion of social justice queries alongside educational topics reflects an awareness of the broader societal implications of AI in academia.
Emphasis on Ethical Considerations: Many queries specifically target ethical concerns, bias detection, and fairness in AI applications, indicating a critical approach to AI adoption.
Practical and Theoretical Balance: The queries span both practical applications (e.g., AI tools for assessment) and theoretical considerations (e.g., AI ethics in research), providing a comprehensive view of the field.
Current and Forward-looking: By including news queries, the method captures both current developments and emerging trends in AI education and social justice applications.
The search queries in this dataset make use of advanced search operators. These operators significantly enhance the precision and relevance of search results. The advanced techniques employed include:
Intitle operator: Queries such as intitle:"chatgpt" intitle:~university focus the search on pages where specific terms appear in the title, ensuring high relevance.
Tilde (~) operator: Used in constructions like ~"Accessibility Enhancement", this operator broadens the search to include synonyms and related terms, capturing a wider range of relevant content.
Boolean operators: The extensive use of AND, OR (represented by spaces), and NOT (represented by minus signs) allows for complex logical combinations of search terms.
Exclusion filters: Numerous -title:"..." operators are employed to exclude irrelevant results, such as calendars, alumni pages, or specific seasons, refining the search output.
Site-specific searches: The site:edu operator restricts searches to educational domains, focusing on academic sources.
Quotation marks: Used for exact phrase matching, ensuring that specific concepts are captured precisely.
Filetype filters: Operators like filetype:pdf target specific document types, useful for finding scholarly articles or reports.
These advanced operators, when combined, create highly specific and powerful search queries. For example, the query for AI Curriculum Development includes multiple exclusions and specific phrase targeting:
~"AI Curriculum Development" ~education -title:"Calendar" -title:"Alumni" -title:"Intern" -title:"About Us" -title:"Upcoming Events" [...]
The queries are designed to navigate the vast sea of online information, zeroing in on pertinent academic and professional content while excluding common but irrelevant pages.